智慧家庭組
SMART HOME GROUP
TARGETS
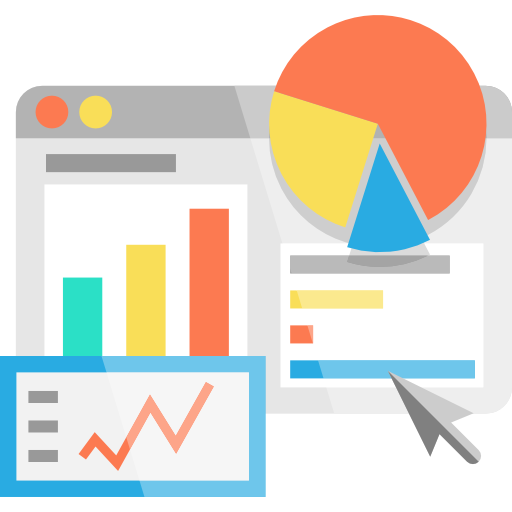
UNSUPERVISED DATA CLUSTERING
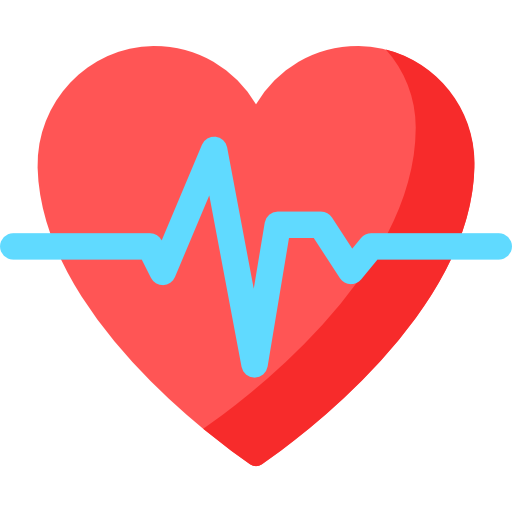
HEALTHCARE SERVICE in SMART HOME
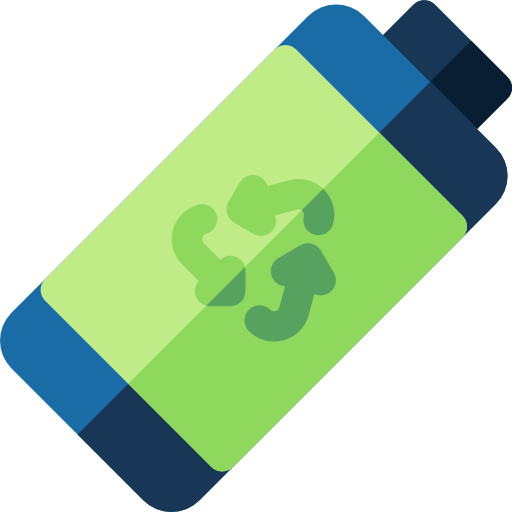
ENERGY SAVING in SMART HOME
RESEARCH
基於文本利用遞歸神經網絡之阿茲海默症快篩系統
A Screening System for Alzheimer’s Disease Based on Speech Transcript Using Recurrent Neural Networks
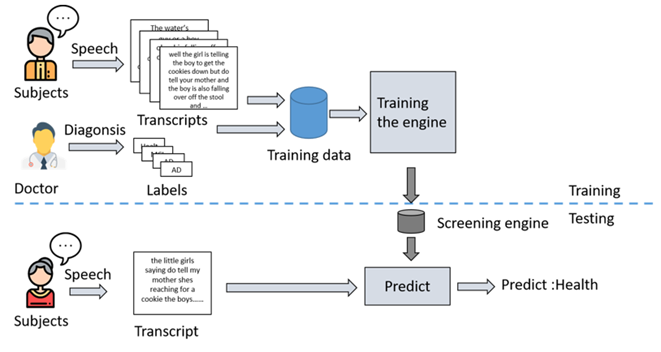
阿茲海默症已然成為世界性的醫療問題,據統計,阿茲海默症已成為全美第六大的死因,也是超過65歲長者的第五大死因,此外,患者數在近年有急遽增長的跡象,亦造成全球醫療的重大負擔。因此,一個得以輔助醫生快速診斷的快篩系統是迫切需要的。在此論文中,我們提出了一個基於語言神經心理測驗的快篩系統,此系統使用詞向量去表示長者測試過程中回答的內容,並使用遞歸神經網絡搭配注意力機制進行分類,比起過去需要萃取語意與語法特徵,並需要額外進行特徵選取的系統,本系統可以更加自動化而不需要專家的協助。使用10折交叉驗證,在區分242筆美國健康長者的作答及257筆患有阿茲海默症的美國長者作答,可以有0.83的準確度;而區分43筆患有輕度認知障礙的美國長者作答及43筆美國健康長者的作答,也有0.71的準確度。測試在各40位的台灣健康及患有阿茲海默症的長者,其準確度甚至可以高達0.89;而區分各30位的健康及患有輕度認知障礙的台灣長者,也能有0.8的準確度。
Alzheimer's disease has become one of the biggest challenges in the healthcare system worldwide. Researches have shown that Alzheimer’s disease is the sixth leading cause of death in the United States and even the fifth leading cause among people aged 65 and older. Therefore, a screening system that can help the doctor to diagnose Alzheimer’s disease is demanded. In this thesis, we proposed a screening system based on the transcripts of speeches spoken by subjects undertaking a neuropsychology test. While most of the related studies have utilized extracted syntactic and semantic features and relied on a feature selection process, the proposed system used word vectors as the representation of a spoken speech, and Recurrent Neural Networks together with attention mechanism as the classifier. Using ten times 10-fold cross validation on an open dataset with 242 speeches samples spoken by healthy controls and 257 samples spoken by subjects with Alzheimer's disease in the USA, a mean accuracy of 0.83 is achieved in our work. And the classification of 43 healthy subjects and 43 subjects with Mild Cognitive Impairment, the model can still achieve 0.71 of accuracy. On the other hand, validate on 40 Taiwanese subjects with AD and 40 healthy Taiwanese subjects, and 30 Taiwanese subjects with MCI and 30 healthy Taiwanese subjects, accuracy of 0.89 and accuracy of 0.8 can be achieved, respectively.
整合使用者行為與深度強化學習之居家即時需量電力管理系統
A Real-time Demand-side Management System Considering User Behavior Using Deep Q-Learning in Home Area Network 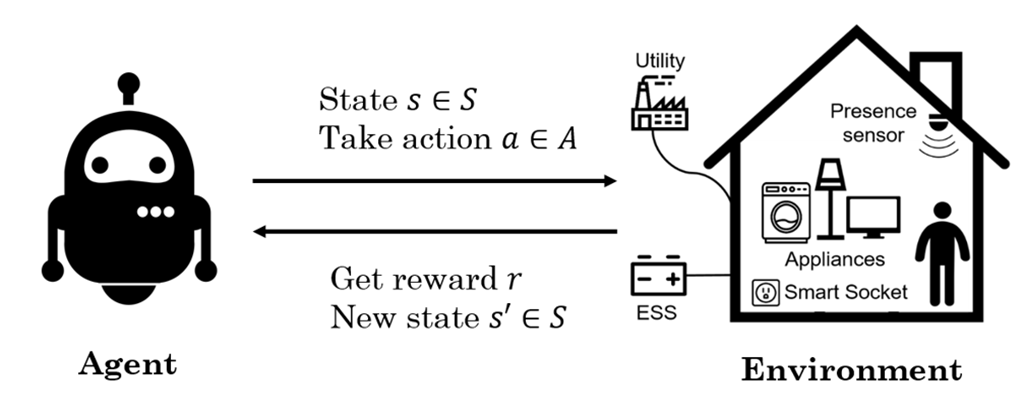
在智慧電網架構中,需量管理系統儼然成為一個重要議題。透過智慧電網與需求端管理,可以在時間電價的機制上通過智能控制和重新調度負載來降低總電力成本,同時降低電力負載的波動性。另一方面,隨著物聯網技術的發展,智慧家庭現在能夠即時監控其家庭狀況並控制能源需求;並且能夠構築自己的家庭區域感測器網路來構建大數據資料庫。隨著近年來電腦計算能力的提高,透過分析大數據資料庫,強化學習等機器學習技術可以很好地應用於需量管理最佳化問題。然而,由於用戶行為和電力消耗的不確定性,仍舊很難確定何為最佳能源管理策略。
在本論文中,提出了一種基於實時多智能體的深度強化學習的方法來解決居家中的需量管理系統問題,並另外考慮了用戶行為以避免干擾用戶舒適度,同時能夠自適應地學習使用者的電器使用偏好並在每日更新中微調系統。在模擬實驗結果中,所提出的需量管理系統提高了智能家居的能源效率,不僅降低了電力成本和峰值,同時降低電力負載的波動性。
In smart grids, demand-side management (DSM) has become an important topic since it can reduce the total electricity cost by smart control and rescheduling of loads, meanwhile, reduce the peak-to-average ratio (PAR) under real-time pricing policy. On the other hand, with the growth of IoT technologies, a smart home can nowadays monitor its household status and control the energy demands; besides, construct their own home area network (HAN) and build the big data database. Thanks to the growing computation ability in recent years, the machine learning skills such as reinforcement learning can be well applied into the DSM problem. However, it is hard to determine a suitable energy management strategy due to the uncertainty of user behavior and the electricity consumption. In the proposed work, a real-time multi-agent deep reinforcement learning based approach has been proposed to solve the DSM problem in HAN, and additionally to consider the user behavior to avoid disturbing user comfort; meanwhile, adaptively learns the appliance usage preference and renew the system day after. The simulation results reveal that the proposed DSM system has improved the energy efficiency in a smart home that not only reduces the electricity cost and peak value but also the PAR value.
基於神經心理測驗與神經網路之數位自動化阿兹海默症快篩系統
A Digital and Automatic Screening System for Alzheimer’s Disease Based on Neuropsychological Test and Neural Network
阿茲海默症以及其他失智症目前不但成為全世界最嚴重的問題,也成爲全世界第五大死因。隨著人口老化,台灣社會已呈現高齡化的結構,失智症患者人數不斷地快速增加,造成家庭照顧者的負擔日益嚴峻。相較於腦影像、血液檢查等較高成本的方式,本研究基於神經心理測驗,透過分析長者所描繪的圖片並利用深度學習的方法,建立一個數位自動化阿茲海默症快篩系統。早期偵測阿茲海默症不但能夠提升他們的生活品質,也能夠減輕照顧者的壓力與照護成本。有鑒於此,本研究利用開放手繪資料集來預訓練神經網路,再將所萃取的特徵與學習到的參數進一步建立數位自動化的阿兹海默症快篩系統,輔助臨床診斷。本研究利用了118位長者紙筆描繪的複雜圖形來區分輕度認知障礙與健康長者,透過一系列的實驗進行驗證後,在ROC曲線面積的指標中達到0.913。另外,本研究也蒐集了60位長者利用數位繪圖板來描繪複雜圖形的資料,在區分阿兹海默症與健康長者實驗驗證後,在ROC曲線面積的指標中達到0.950的結果。
Alzheimer’s disease (AD) and the other types of dementia have become one of the most serious global health issues and the fifth leading cause of death worldwide nowadays. Therefore, early detection of the disease in the stage of mild cognitive impairment (MCI), which is a prodromal stage of progressing to AD and mild AD, is crucial in order to improve the quality of life of the patients and to decrease the burden of their caregiver and clinicians. The aim of our study is to design a digital screening system based on the Rey-Osterrieth Complex Figure (ROCF) neuropsychological drawing test in order to assist the clinicians to detect whether the subject is MCI or AD against healthy control (HC) automatically. A data-driven deep learning approach is implemented in this work for building the screening system. An architecture of convolutional neural network is designed for pre-training and extracting useful features from the figures drawn by the subjects. The learned features are then transferred to our collected dataset for further training of the classifier in order to distinguish the patients with MCI or AD against HC. As a result, a mean area under the receiver operating characteristic curve score (AUC) of 0.913 is achieved for classifying MCI vs. HC in traditional pencil and paper based ROCF called NTUH_ROCF dataset. On the other hand, dataset that collected using digitalize graphics tablet and smart pen based which is called NTUH_D-ROCF achieved 0.950 of AUC in classifying AD vs. HC.
基於神經心理測驗利用遞歸神經網路與特徵序列所開發之阿茲海默症語音評估系統
A Speech Assessment System for Alzheimer’s Disease Based on Neuropsychological Tests Using a Novel Feature Sequence Design and Recurrent Neural Network
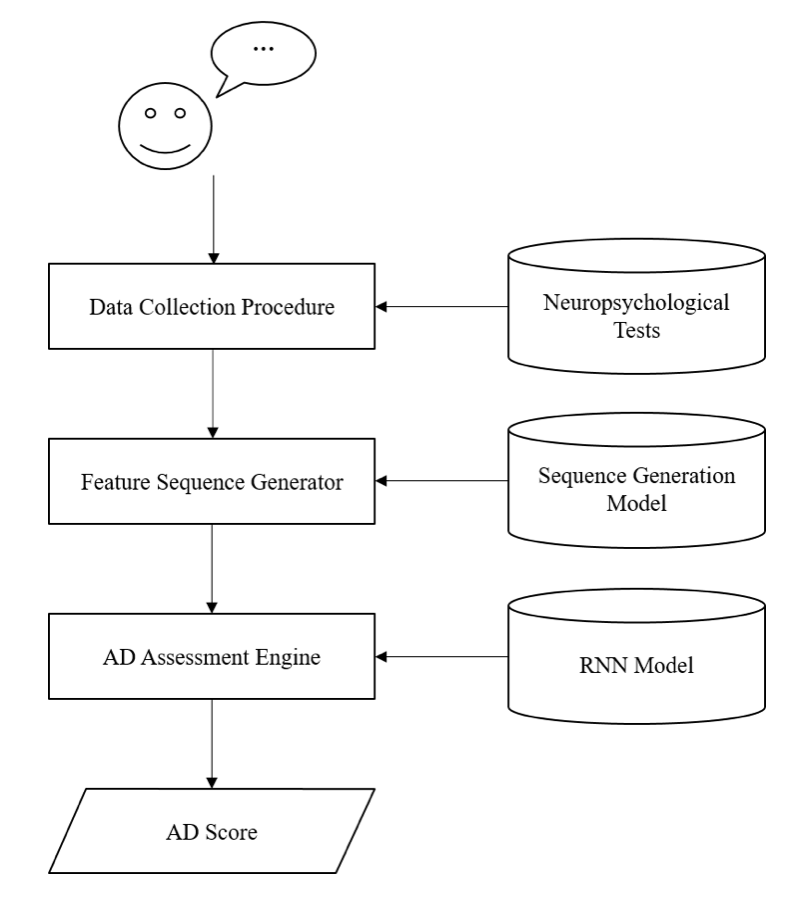
在這項研究中,我們提出了一種新的特徵序列,用於表示阿茲海默病患者在進行神經心理測驗的語音,並利用雙向遞歸神經網絡進行分類。在研究中我們也提出一個基於深度卷積遞歸神經網絡的特徵序列生成器,實驗證明特徵序列可以藉由該生成器自動生成。交叉驗證總共120個樣本,其中一半來自認知健康受試者,其他來自阿茲海默病受試者,接受者操作特徵曲線 (AUROC) 可以高達0.838。
In this study, we have proposed a novel Feature Sequence representation for characterizing speech data from patients with Alzheimer’s disease in a neuropsychological test scenario. An Alzheimer Disease Assessment Engine based on a bidirectional recurrent neural network with gated recurrent unit is trained to carry out the classification. Moreover, we have shown that the Feature Sequence can be generated automatically with the help of the Feature Sequence Generator, which is based on a deep convolutional recurrent neural network trained with the connectionist temporal classification loss. Cross validating with a total of 120 samples, which half of them were from the cognitive healthy subjects and the others were from the Alzheimer’s disease subjects, an area under the receiver operating characteristic curve (AUROC) score of 0.838 is achieved.
使用者導向於動態共享價格機制之住商混和型社區需量反應電力管理系統
User-driven Demand-side Management in Mixed-use Community under Dynamic Sharing Price Mechanism using Particle Swarm Optimization
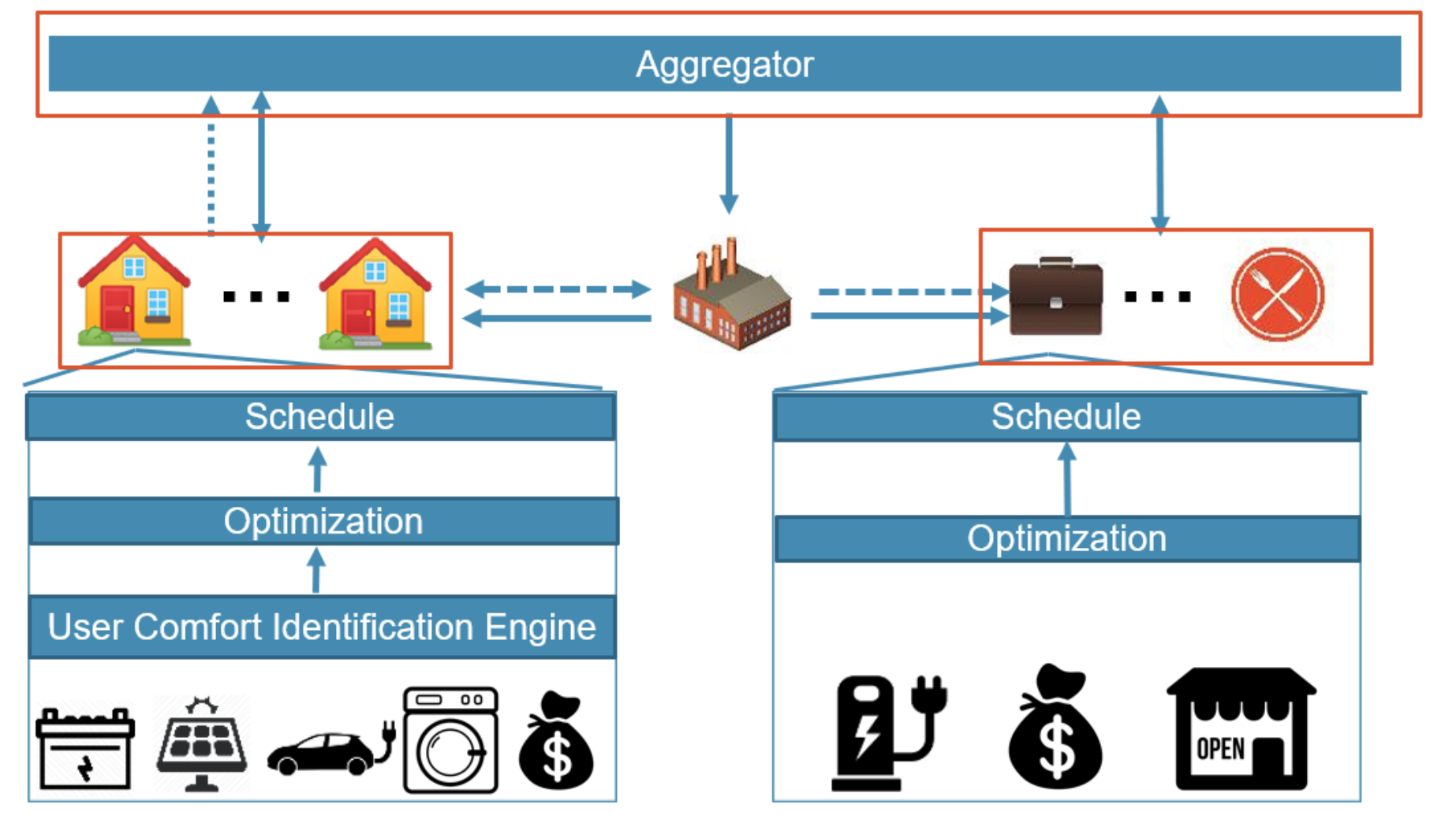
在時間電價的機制下,透過需求端管理能使用戶可以更加有效的使用能源,而電動車以及小型的住宅型發電裝置蓬勃發展也讓住宅社區可運用的能源選項更加多元。為了能夠更有效的使用能源,我們提出一個整合住宅區與商業區的使用者的電力管理系統。透過整合用電習慣較為彈性的住宅區使用者以及用電習慣較為固定的商業區使用者,整體社區的用電品質及用電的效率可以得到顯著的提升。在實驗結果當中,住商混合的住宅區較純住宅區節省了44.24%的電費、商業區的辦公室節省了22.39%的電費,而商業區的餐廳也節省了13.66%的電費。
Demand-side management ability in a residential area has been improved a lot under the enforcement of real-time pricing mechanism. In order to utilize the energy efficiency in a residential area and improve the energy quality in whole community. In this work, we aim to integrate the residential users and commercial users into one DSM system. By integrating them, we can utilize the energy more efficiency by sharing the surplus energy in a residential area to the commercial area. The result shows that, in the residential user, the cost is 44.24% than purl residential community on average. In addition, the commercial user also reduces the energy cost. The office user reduces 22.39% and the restaurant user reduces 13.66% on average.
基於智慧家庭中的動作感測器開發失智症輔助偵測系統
A Supporting System for Quick Dementia Screening Using PIR Motion Sensor in Smart Home
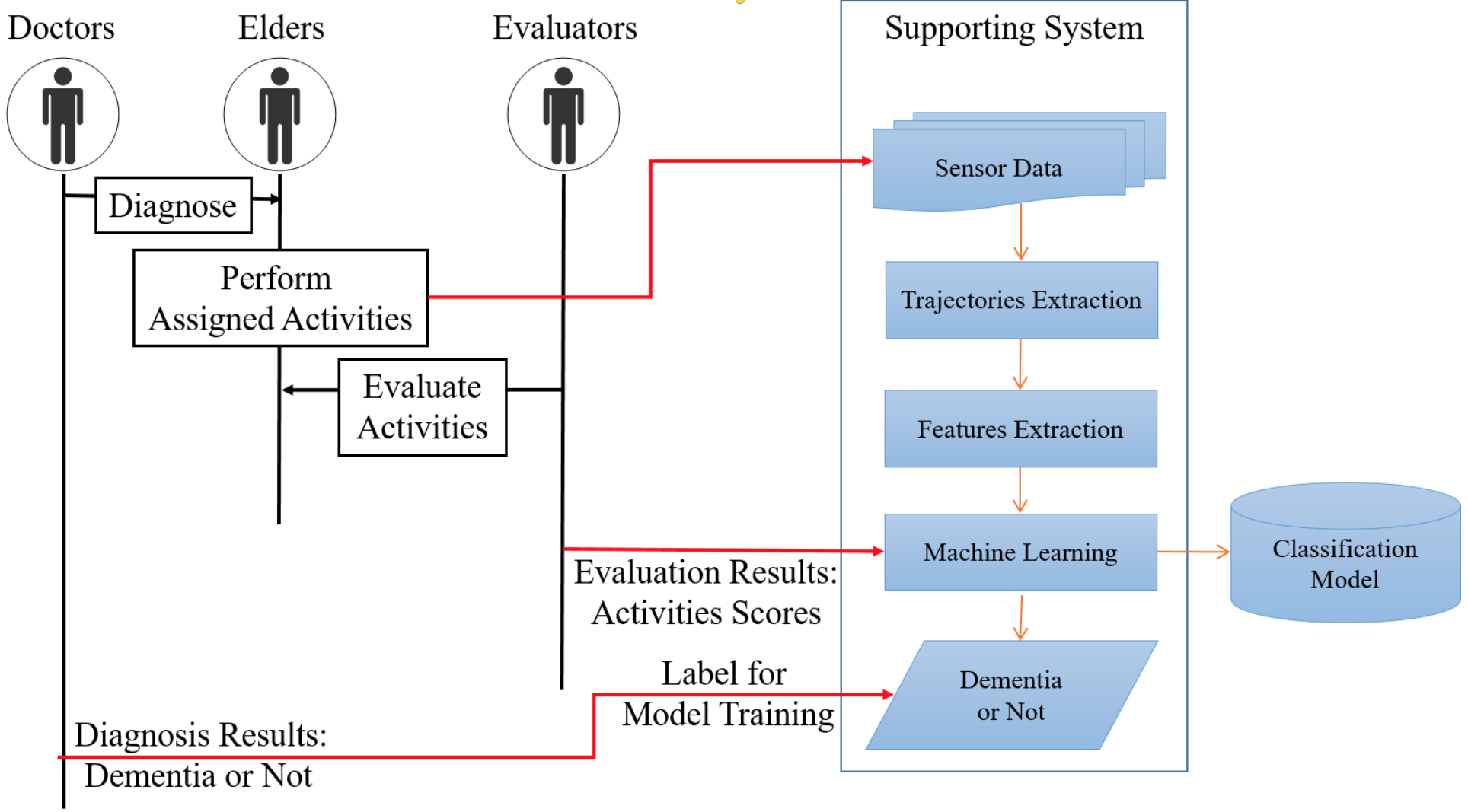
我們提出了一個輔助系統,可以根據長者行為能力,在2到4小時內預測長者患有失智症的可能性。在測試過程中,長者只需要在智慧家庭的環境中執行從工具性日常生活活動(IADL)中選擇的某些活動,系統將藉由環境中裝設的活動感測器去抓取長者的移動軌跡,並且分析是否有漫遊的潛在相關性。基於研究提出的漫遊特徵,我們利用機器學習來執行分類任務,並測試在兩組資料集上。兩個資料集分別有232位長者,包含7位失智長者;以及我們自行收集的資料,包含30位長者,其中9位患有失智症。在第一組資料集上,系統的平均精度和召回率均高達98.3%,ROC曲線下面積(AUC-ROC)則為0.846;在第二個資料集的平均精度和召回率則分別為89.9%和90.0%,其中AUC-ROC為0.921。
We proposed a supporting system that can quickly estimate the likelihood for an elder of having dementia based on 2 to 4 hours monitoring of a behavioral test done by the elder. During the test, the elder only needs to perform certain activities selected from the Instrumental Activities of Daily Living (IADL) in a smart home environment, and their movement trajectories will be extracted from motion sensors and be analyzed to find potential correlation with the indoor wandering patterns. A machine learning algorithm is selected to carry out the classification task, based on our proposed features of the wandering patterns. Two datasets are employed for performance evaluation, where the first one is 232 elders including 7 dementias, whereas the second one is collected by ourselves from a senior center, which is 30 elders including 9 dementias. It turns out that the average precision and recall for the first dataset are both up to 98.3% with Area Under the ROC Curve (AUC-ROC) being 0.846, and those for the second dataset are 89.9% and 90.0% with AUC-ROC being 0.921.
基於異質數據及智慧家庭之無參數活動辨識系統
Nonparametric Activity Recognition System in Smart Homes Based on Heterogeneous Sensor Data
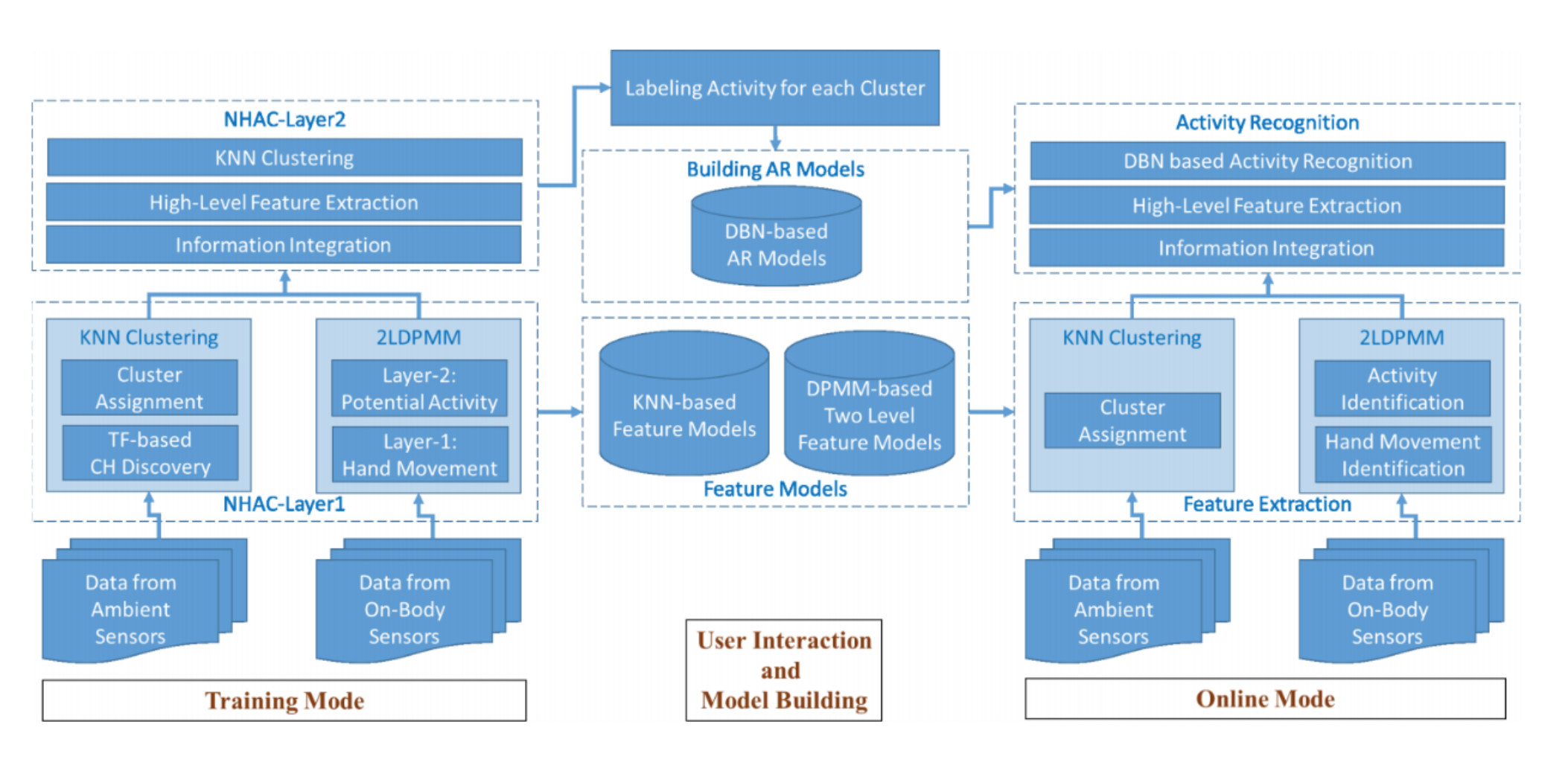
我們為智慧家庭中獨立生活的長者提出了一種活動辨識(AR)系統,以實現“老化到位”的概念。研究所提出的活動辨識模型通過整合來自環境感測器和穿戴式裝置的異質數據來識別日常生活活動(ADL)。另外,研究提出的系統採用無參數的方法,這使得系統不需要花費過多的人力去訓練模型。研究結果顯示,該系統在辨識活動上,平均精度和召回率分別高達98.7%和99.0%,足以顯見其相當適合於真實的情況下,觀測長者的活動狀況。
We proposed an activity recognition (AR) system for the elder living independently in smart homes to achieve the concept of “aging in place.” The AR model adopted by the proposed system is powerful to recognize meaningful Activities of Daily Living (ADL) by integrating heterogeneous data from both ambient and on-body sensors. Moreover, the proposed system adopts a nonparametric approach, which requires much fewer efforts from humans. The average AR precision and recall rates of this proposed system are up to 98.7% and 99.0%, which indicates its feasibility of deployment in a real-life home environment for monitoring users' ADL with promising performance.
整合電動車與再生能源並落實共享經濟於住宅型社區之電力需求管理系統
Demand-side Management in Residential Community Realizing Sharing Economy with Bidirectional Plug-in Electric Vehicle and Renewable Energy
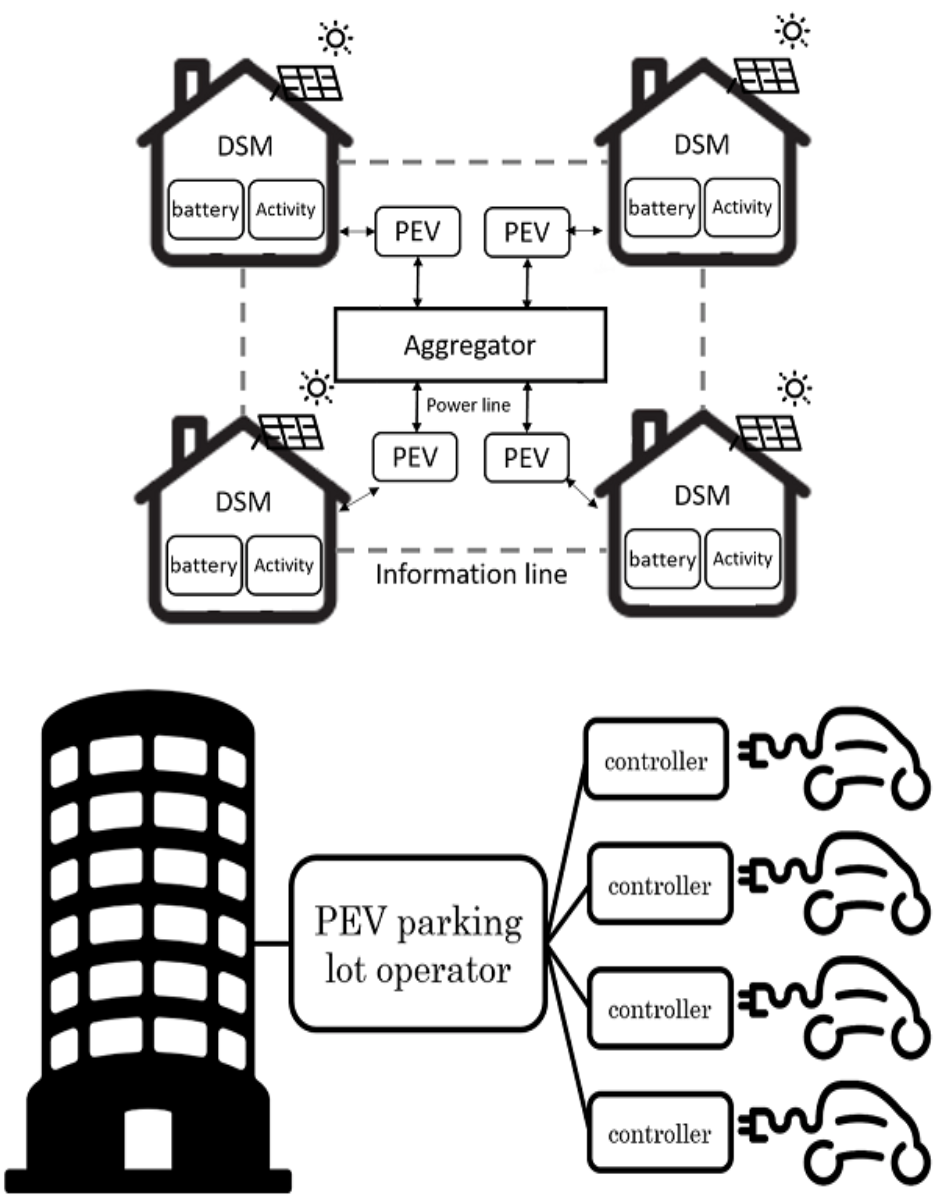
透過智慧電網與需求端管理,不但可以有效減少家中用電支出,還能同時降低電力負載的波動性。當需求管理系統推及到社區時,我們設計了一個公平的電動車共享系統,以節省社區的電力支出及電力負載波動。在研究中,我們同時考慮電動車、再生能源、電力儲存裝置之家庭需量管理排程最佳化。更進一步於商辦大樓結構下,提出多輛電動車充放電控制排程最佳化以降低大樓電力支出。若電動車停靠時有著較低的電量,系統可以排程各電動車的充電時間,以降低負載並可以節省43.3%的電費。
In smart grids, demand-side management is one of the important function since it reduce the total electricity cost of each customer. On the view point of a community, we design a fairness strategy to share Plug-in Electric vehicles' battery with neighbors to reduce the total electricity cost and peak to average ratio. In our problem formulation, each home is assumed to be connected to a renewable energy resource, be equipped with an energy storage device, and have an optional Plug-in Electric vehicles with the vehicle to grid ability, and the formulation is in terms of a multi-objective optimization cooperative game to facilitate power sharing among negihbors. In the results, if Plug-in Electric vehicles come with a lower battery state, system reduce 43.3% of electricity cost.
在少量標註資料下基於增強對比的高效半監督學習之文本分類
Contrastenhanced Effective Semisupervised Text Classification with Few Labels
文本分類是一個自然語言處理中非常重要的問題。傳統的文本分類需使用大量的標記數據或是額外的翻譯模組來訓練深度神經網路才能達到好的分類效果,然而這在許多實務問題上並不可行,例如資料難以大量取得、標記資料成本昂貴或難以獲取。這樣使得訓練深度神經網路在文本分類任務上變得十分困難。相比之下,無標記資料則相對易於取得,例如網路上的公開資料、且因不需要標記而降低收集成本的資料等。故在本論文中我們提出了一個基於自我訓練的辦監督學習演算法。我們根據資訊理論的指標選擇了適當的無監督資料來提升模型表現,並透過對比學習更有效的利用資料並避免過擬合的問題,也特別抑制了訓練中可能有的誤差傳播問題。透過實驗證明,在每類只有 10 個標籤的樣本的情況之下,同時又無需其他額外的模組,我們的演算法在準確性上超過了原本只用少量標註資料訓練的 BERT 模型 13.4%。同時,我們的演算法在只用了每類 10 個標注資料的情況下,其性能逼進了用數以千計的標記數據訓練的全監督學習的表現,與之只相差 3.4% 的準確率,並且優於以往最新技術的準確率 3.6%。
Traditional text classification requires thousands of annotated data or an additional Neural Machine Translation (NMT) system, which are expensive to obtain or access in real applications. In this thesis, we present a ContrastEnhanced Semisupervised Text Classification (CEST) framework under labellimited settings without incorporating any additional NMT system or data augmentation process. First, a certaintydriven method is employed to select appropriate unlabeled data for selftraining. Then, a reliable similarity graph is proposed to induce the smoothness among data instances. Finally, the training is formulated as a“learning from noisy labels"problem, which is then optimized accordingly. A salient feature of the formulation is that it explicitly suppresses the severe error propagation problem in conventional semisupervised learning. With solely ten labeled data per class, the performance of CEST falls within the 3.5% range of that of the fullysupervised pretrained language models finetuned on thousands of labeled data while outperforming the previous stateoftheart algorithms in the literature by 3.6%, without incorporating any additional systems.
嵌入停頓編碼之具對比增強的認知障礙自動檢測系統
Contrastenhanced Automatic Cognitive Impairment Detection System Embedded
with Pause Encoding
隨著全球老年人口的逐年增長,阿爾茨海默病患者也同樣地增加,現有的醫療保健系統由於患者對治療和早期診斷的高需求導致相當大的負擔,因此,對認知障礙篩查系統的研究被廣泛關注以協助醫生診斷阿爾茨海默病去降低負擔。在本論文中,我們提出了一種基於自動轉錄的嵌入停頓編碼之具對比增強的認知障礙自動檢測系統。對於認知障礙,語音中的停頓模式是一種常用的聲學特徵,可以提供更多信息給予模型去進行更好的判斷,此外,反向翻譯和對比學習將使我們的對比增強模型在隱藏空間上有更好的表示,對比模型在微調具暫停嵌入的轉錄後可用來檢測患者的認知障礙。為了提高所提出之系統在現實世界中的適用性,我們的系統是全自動的,並且可以生成可以解釋的的結果,我們也使用英語和中文兩種語言評估我們的系統,兩種成功的結果都證明了我們系統的多語言能力。在對我們工作的定量評估方面,我們的系統在 ADReSS 數據集上自動檢測阿爾茨海默病可以達到 81% 的準確率,同時。此外,我們的系統在解決檢測輕度認知障礙(介於健康和阿爾茨海默氏症之間的中間階段)這一更具挑戰性的任務方面的準確性亦有不錯的表現。我們亦擴展檢測輕度認知障礙的任務到更非結構化語音的數據集,也就是我們於本地端收集的自傳式記憶數據集上,我們的系統的準確率平均可以達到 71% 準確率。
As the global elderly population grows annually, healthcare systems face a burden from the rise in Alzheimer’s patients due to its high demand for treatment and early diagnosis. Therefore, research on cognitive impairment screening systems is studied widely to assist doctors in diagnosing Alzheimer’s disease. In this thesis, we propose a contrastenhanced automatic cognitive impairment screening system embedded with paused encoding based on automatic transcription. For cognitive impairment, the pause pattern in speech is a commonly studied acoustic feature that can provide more information based on which the model can make a better distinguishing judgment. Moreover, backtranslation and contrastive learning represent a better contrastenhanced model. After finetuning the transcripts embedded with pause, such a contrastenhanced model is applied to detect the patients’ cognitive impairment. To improve the applicability to the real world, our system is fully automatic, and its generated results can be shown to be explainable. We evaluate our system in two languages, English and Chinese, and both successful results demonstrate the multilingual ability of our work. In terms of quantitative evaluation of our work, our system can achieve 81% accuracy while automatically detecting Alzheimer’s disease on the public ADReSS dataset. Besides, the accuracy of our system in tackling a more challenging task of detecting mild cognitive impairment (MCI), the middle stage between healthy and Alzheimer’s, is highly promising. As for the same task of detecting MCI, on a more unstructured speech dataset, called autobiographical memory dataset collected locally, we show that the accuracy of our system can reach 71% on average.